Fantastic Info About When To Use Arma And Arima Multiple Trend Lines Excel
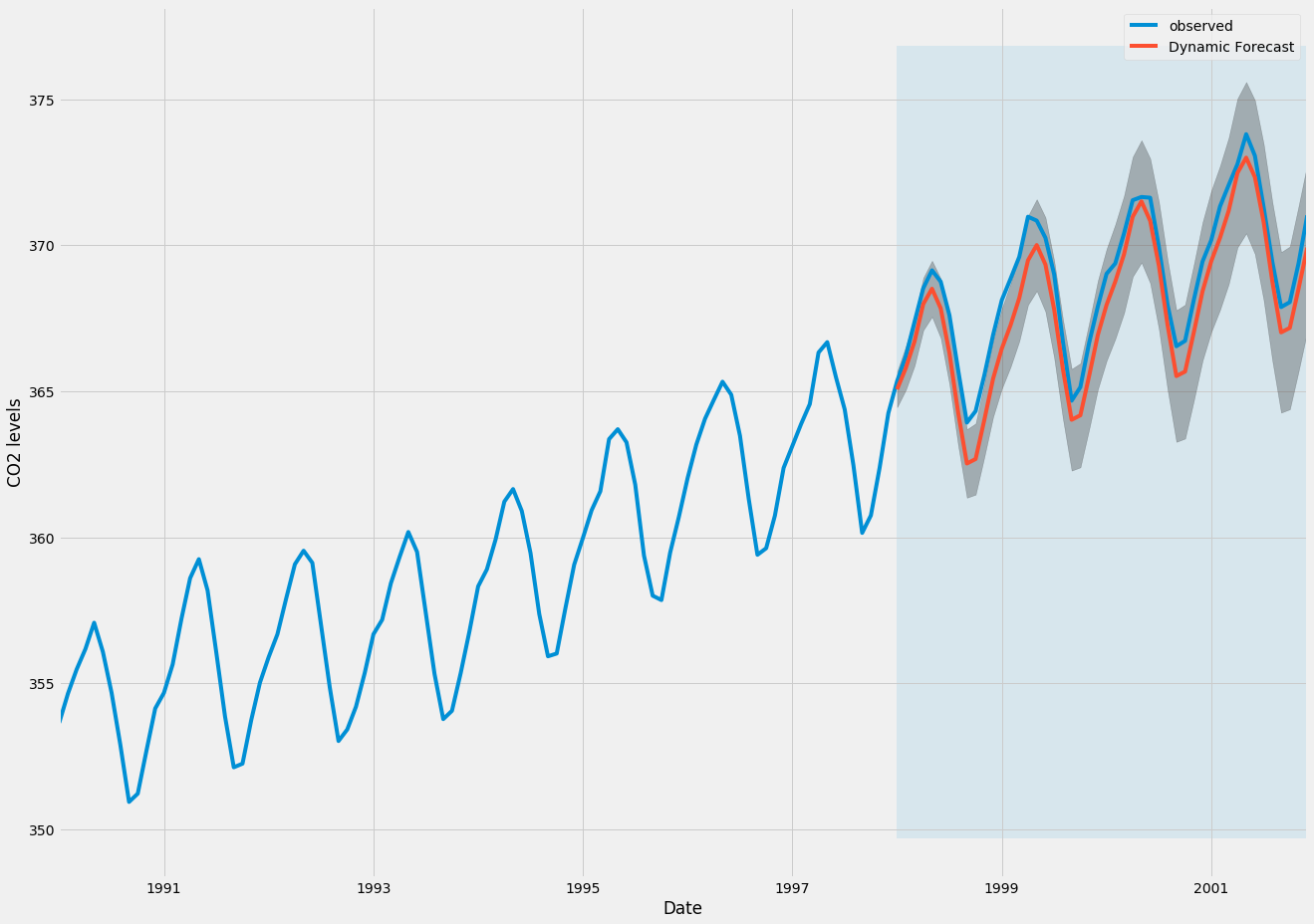
Estimation in an arma( ) model—estimating the coefficients 1;
When to use arma and arima. To incorporate this complex nature of time series data into models, box and jenkins introduced the autoregressive integrated moving average (arima) model. Time series analysis comprises methods for analyzing time series. The choice between arma and arima models depends on the behaviour of the time series data.
Predicting the future has forever been a universal challenge, from decisions like whether to plant crops now or next week,. Time series data is a collection of observations in regular, defined intervals over time. Time series analysis is used in a wide variety of.
Photo by dastan khdir on unsplash. If the data exhibit stationary. Some common examples of time series data are monthly sales metrics, daily stock prices, etc.
Model and predict the dependence structure of the errors. See what others are saying. Steps for time series analysis.
Q in (16)—is in general fairly complicated. Time series forecasting focuses on analyzing data changes across equally spaced time intervals. Much more so than in linear regression.
Components of time series data. When to use which model? 1 what are arima and arma models?
In an arima model, the integrated part of the model includes the differencing operator (1 − b) (where b is the backshift operator) raised to an integer power. In time series analysis, the most highly used models are ar(autoregressive), ma(moving average), arma(autoregressive moving average),. To better comprehend the data or to forecast upcoming series points, both of these models are fitted to time series data.
In this article, i will. In statistics and econometrics, and in particular in time series analysis, an autoregressive integrated moving average (arima) model is a generalization of an autoregressive moving average (arma) model. A time series is a sequential set of data points, measured typically over successive times.
Exploratory data analysis and transform data into stationary data. Now, we will combine both methods and explore how arma(p,q) and arima(p,d,q) models can help us to model and forecast more complex time series. The arima (auto regressive integrated moving average) model is an extension of the arma model, with the addition of an integration component.
The arma model is used to describe time series data that is stationary, meaning its statistical properties do not change over time.